Enterprises invest in artificial intelligence (AI) innovation for competitive advantage. New AI models promise increased productivity and exciting new possibilities. But hidden costs often derail the budget and raise questions about financial sustainability. It may even push the enterprise to bankruptcy.
Here are the not-so-obvious expenses related to AI adoption.
Cloud costs
Organisations now use AI extensively, especially GenAI, for innovation. Such AI-powered innovation enables advanced analytics, hyper-automation, and several other use-cases.
The effectiveness of these applications depends on fast and scalable delivery channels.The cloud offers unlimited processing power and storage. But most organisations do not realise that AI Infrastructure is much more resource-intensive than traditional workloads. Innovation based on large language models (LLM) demands huge computing resources. Also, GenAI computing requirements are unpredictable. They are often multi-layered and cost up to five times higher than traditional cloud services.
Most organisations also underestimate the cost of essential hardware such as GPUs and TPUs, that power AI based applications. The prices of these hardware have shot through the roof of late. The demand brought about by GenAI and the post-COVID supply chain disruptions have resulted in steep price hikes.
As the AI system grows and demands more resources, cloud expenditures spiral out of control and become a budget black hole. Underestimating these costs often forces organisations to retreat from the cloud. Gartner estimates that more than half of the organisations abandon their AI attempts owing to their missteps in cost estimation and calculation.
To keep AI cloud and infrastructure costs in check, observe and analyse resource consumption and performance of AI models. Use observability platforms, such as Dynatrace, to track the performance, behaviour, and cost of AI models and services.
The Dynatrace platform integrates with cloud services to offer end-to-end operational views. It monitors infrastructure data, including memory utilisation, temperature, and process usage. It also integrates with custom models such as Google Tensor Processing Unit, Amazon Elastic Inference, and NVIDIA GPU. Such integrations offer comprehensive tracking regardless of the stack. Users can develop dashboards to integrate logs, metrics, problem analytics, and root-cause information.
Energy costs
Many enterprises factor only the cost of building AI models. Running AI applications is equally cost-intensive.
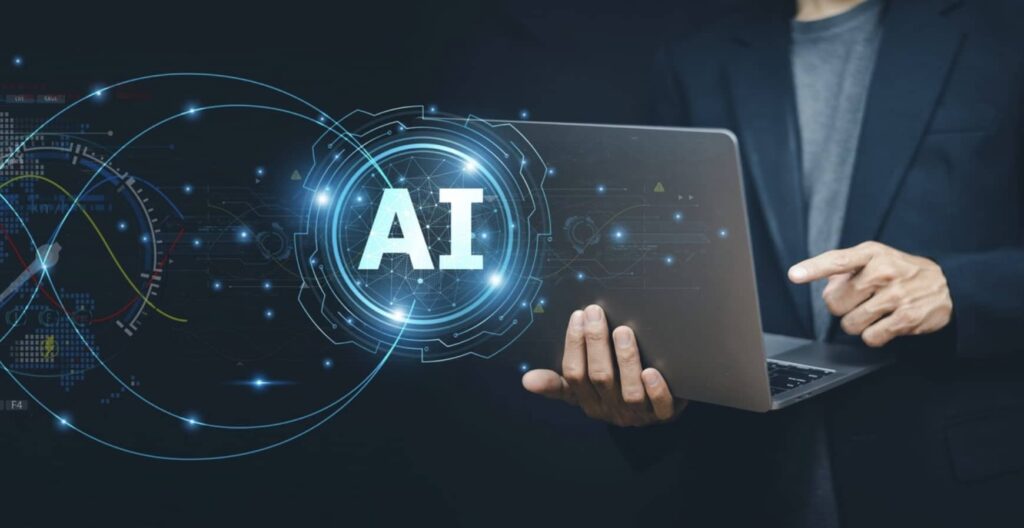
The energy required to run foundational AI models is a big cost sinkhole. Training AI models involves huge energy consumption. To put things in perspective, it takes eight current-generation GPUs five days to fine-tune a 70B parameter model. The process requires approximately 1308kWh of power over five days. Even if organisations factor in these expenses, they underestimate the recurring or repetitive costs. AI models need continuous monitoring and refining, adding or duplicating the costs. These costs multiply in innovation involving several experimentation and proof-of-concepts. Often, innovation involves several decentralised pilot projects that fail but become part of the learning curve. These initiatives entail significant sunk costs
The way the AI models function can add to the costs. For instance, GenAI enables large, parallel computations and increases speed. But it creates an extra layer of technical debt and increases energy consumption.
Leading AI models burn through $10 million per month or more in power costs to operate at scale. Even such a high resource use is often insufficient, forcing companies to throttle access and limit consumption.
The energy needed to power data centres for commercial AGI does not exist yet. In the meantime, power-hungry AI companies impose high API costs to recoup their massive energy bills.
Transparency helps to overcome cost shocks once the AI models become operational. Transparent AI makes explicit the technical details and inner workings of the AI models. The spin-off benefit is better compliance and lesser risk of costly errors.
The Dynatrace platform offers visibility into every layer of the application AI stack. Complete visibility helps optimise costs. It also enables proper attribution by tying costs to specific business functions or customers.
Data costs
Many organisations also underestimate the data costs associated with AI.
The efficacy of any AI system depends on the data fed into it to train the algorithms. Without relevant and current data, the underlying algorithms deliver faulty results. Collecting accurate and relevant data depends on sifting through mountain loads of information. The short-listed data also needs cleansing and tagging. The process is time-consuming and costly. Data procurement, management, storage, and security costs add up.
Also, most companies developing AI systems have been scraping massive amounts of copyrighted data from the web. The defence of “fair use” is not tenable once the AI models built using such data take on commercial use. Most companies have not yet considered the costs of licensing training data from copyright holders. The possible penalties for copyright infringement will be even higher once the ongoing lawsuits end.
The macro-level solution is to plan and budget well. To understand the financial footprint, factor in data acquisition, management, and possible liability costs.
Here again, tools such as Dynatrace come to the rescue. At an operational level, Dynatrace’s AI observability features pinpoint bottlenecks and inefficiencies. Such insights identify redundant computations and unnecessary data processing steps, which drain costs.
Operational costs
Most organisations running AI may take cognisance of infrastructure and energy costs. But they still underestimate some operational costs.
AI is not a perfect science. LLMs often hallucinate and generate incorrect or nonsensical responses. Such errors can lead to huge financial losses and reputational damage.
The solution is to create robust Retrieval Augmented Generation (RAG) pipelines. RAG detects model drifts. In doing so, it augments prompt responses with data retrieved outside the LLM. Such an approach overcomes the knowledge gaps in the training data and reduces hallucinations.
Dynatrace offers insights into the RAG architecture from both retrieval and generation aspects. It leverages semantic caches to detect model drift in embedding computations.
Dynatrace AI observability offers a granular understanding of the AI stack, including hidden costs. It offers detailed workflow analysis, resource allocation, and end-to-end execution insights. Users get visibility into infrastructure utilisation, performance, and application health. Such insights make it easy to safeguard against waste and unexpected costs.
AI becomes untenable when costs outpace the value delivered. Acknowledging the hidden and unforeseen costs of AI adoption is the first step towards addressing them. A proper cost perspective enables correct pricing. AI becomes a springboard for innovation instead of a financial sinkhole.