AI-powered data management systems promise businesses great insights from their raw data. But almost all AI adopters encounter data-related roadblocks.
Most businesses accumulate data. But only a few succeed in deriving tangible and sustained insights from it. Here are six ways to get the most from AI-powered data management tools.
Access to Wider Data Sources
Effective business insights depend on capturing all types of data, including unstructured data.
Traditional data analytics tools process only structured data, such as customer databases and bank statements. This worked in the past, when the bulk of the data consisted of such organised data.
But of late, the nature of enterprise data is changing. The bulk of business data is now unstructured data, such as social media posts, images, emails, and more. Structured data constitutes only a small fraction of business data. Any analytics based on only structured data will generate insufficient or limited insights.
The emergence of large language models (LLMs) equips business intelligence (BI) platforms to process unstructured data. A business can, for instance, use BI tools to track sentiments from social media comments.
The latest BI tools automate data ingestion, transformation, and loading processes to make analytics more efficient and comprehensive.
Improved Data Discovery through Metadata Management
Business data comes from various disparate sources and piles up organically. Such data is often inconsistent, and some valuable data remains trapped in silos. It becomes difficult, time-consuming, and costly to identify relevant data from such an environment.
Intelligent metadata management improves data discovery amidst such challenges.
Metadata provides context, meaning, and structure to raw data. Most businesses do not understand the importance of metadata and, hence, do not spend time adding metadata to their data. Insufficient metadata information makes data discovery difficult.
Data management tools such as Informatica enable enterprises to manage metadata. Informatica’s data lineage capabilities provide complete visibility into the data. It makes explicit where the data originates and its journey through various processes.
These insights make it easy and less time-consuming to identify relevant data. It also offers work teams the context behind the data, enabling them to make better data-related decisions.
When fed with relevant and up-to-date data, the integrity of the AI algorithms improve.
Improved Data Quality
Poor data quality can wreck the best-laid AI strategies. Training and operating genAI models especially need large volumes of high-quality data.
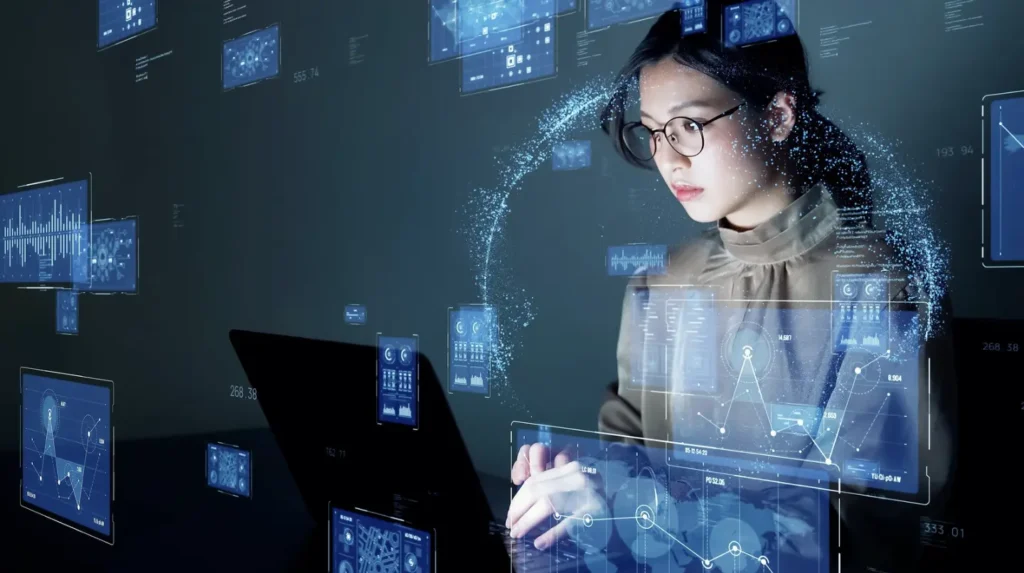
The definition of quality is subjective. But a common ground for quality clearing data of errors and inconsistencies. Integrating inconsistent data for analytics is difficult. Even if integrated, the results may skew in unpredictable ways. Inconsistencies can introduce inaccuracies and bias into the output.
Many enterprises underestimate or ignore the difficulties of cleaning, labelling, and organising data. The process is time and resource-intensive. The costs add up quickly.
And contrary to popular perception, ensuring data quality to train algorithms is not a one-off task. Machine Learning models come with the risk of accuracy drift over time. Often, the model retrains itself based on new and changing data inputs. The effectiveness of the algorithms over time depends on controlling the drift by retraining the model.
The solution lies in automated data cleansing and cataloguing. Automation does routine data cleansing and integration tasks in a jiffy. It reduces manual tasks and decreases labour costs.
Automated Data Governance and Compliance
AI-based systems suffer from trust issues. The tendency of AI algorithms to hallucinate makes the output unreliable. For critical business systems, even a small hallucination can have devastating implications.
Effective data governance allows business users to control the data and establish trust.
Effective data governance:
- Improve access to data. Data governance lays down rules and protocols to access data. Such rules also democratise data access across the board.
- Makes it easy to classify and protect sensitive information. Data governance policies define sensitive data and establish access control rules for such data.
- Ensures data privacy. One of the main drivers of differentiation today is personalisation and experience management. But data privacy often comes in the way of such initiatives. Data governance enforces security policies, controls, and data loss prevention measures. Such measures ensure privacy even while enabling access to authorised personnel.
- Ensures compliance with regulations. The increasing use of data by AI applications has triggered more and more regulations around using data for AI. Regulations such as EU GDPR and US HIPAA already impose several responsibilities on data handlers. The increasing stakes of data have led to new regulations, such as the EU AI Act and the Biden Administration’s Executive Order.
Using Natural Language Processing for Data Exploration
Until not too long ago, business users relied on data scientists to derive insights from data. In today’s fast-paced world, where data has a short shelf-life, such reliance often becomes a bottleneck. By the time business users get the insights, the value of such insights would have become diminished.
GenAI enables conversational or natural-language-based UI/UX in enterprise BI platforms. Such capabilities empower business users to ask questions in natural language. They get instant insights as well. Users no longer have to depend on experts to derive actionable insights from data.
Top AI-powered data management platforms analyse data beyond the user’s query. It provides natural language explanations to plug remaining knowledge gaps.
Better Data Architectures
In most businesses, the data architecture develops organically over time. This makes the architecture complex and riddled with silos. AI-powered BI platforms offer users a simple front end that makes the data more accessible.
AI-powered BI platforms:
- Offer comprehensive data discovery and mapping capabilities. Efficient data discovery helps business users make sense of the data type, location, format, and usage.
- ·Centralise data. A centralised data store removes redundancies and duplications. It also delivers to users a consistent experience across all data types.
- Automate code generation and validation capabilities. Gen AI-powered BI platforms come with capabilities to write SQL or Python code to fix data architecture. Businesses can leverage automated coding capabilities to make validation checks and automate cleansing.
- Improve visibility and transparency. BI platforms offer stakeholders a clear view of the data processing pipeline to ensure the accuracy and reliability of data. Previously, ensuring accuracy depended on ad-hoc measures. The user, for instance, had to rely on someone with previous knowledge of the data.
- Deliver automated semantic layer enrichment capabilities. The latest BI platforms leverage genAI to add context to the data. It can, for instance, add a data type to numeric data fields or generate user-friendly descriptions to a data set.
Businesses can leverage AI-powered BI platforms to reimagine their data strategy. They can, for instance, set up centralised data marketplaces with verified data.
Consider a bank. The data management tools make the data more accessible, actionable, and auditable. Bankers can leverage the relevant information to process credit approvals in double-quick time.
It requires a robust data management platform for enterprises to leverage the power of AI and make better use of their data. One platform that serves these purposes is Informatica’s Intelligent Data Management Cloud platform.
The platform applies automation to classify, govern, and protect data.
Advanced querying and quality tools automate processes that would otherwise take months.
The platform breaks down silos and brings data from source systems to a centralised data lake. In the process, it makes data operations more visible and transparent. Advanced data mesh architectures speed up data ingestion and processing.
Businesses get greater control over the data. Stakeholders get a more complete picture of the information they need to power their decision-making. Data analysts can tap into these data assets to build analytical models that further decision-making.
As a case in point, Informatica enables banks to ingest financial and customer data 50% faster. It also reduces predictive model design and maintenance times by 60 to 70%. The platform accelerates the end-to-end process by a whopping six months!
Traditional data management systems struggle to keep up with the rapid expansion of data volume, variety and velocity. But the latest AI-powered BI platforms such as Informatica are up to the task. These platforms become a powerful arsenal in the hands of businesses, to seize competitive advantage.