As competition intensifies and the environment becomes increasingly uncertain, businesses look at predictive analytics tools for insights to improve performance.
Predictive analytics combines historical data, statistical algorithms, and machine learning to predict outcomes. Plain-vanilla analytics describes the data and finds the patterns inherent in the data. Predictive analytics go beyond and use such insights to confirm the likelihood of what will happen in the future.
Here are seven powerful predictive analytics tools and platforms that are becoming popular in 2023.
1. RapidMiner Studio
RapidMiner has a strong reputation in data and text mining. Of late, the platform has added predictive analytics capabilities. RapidMiner Notebooks make the development of predictive analytics models simple.
The easy GUI and the integration of data processing and modelling tasks make it easy for non-technical users to perform complex data analyses.
The key USPs of RapidMiner include
- Ability to leverage Rapid Miner’s core capabilities of extracting data from diverse sources and integrating it into predictive modelling workflows. Integrated tools make data preprocessing, cleansing, transformation, visualisation, and modelling easy.
- Augmented capabilities for data preparation, model generation and model deployment.
- Easy-to-use, drag-and-drop interface for building predictive models. Users may set up machine learning algorithms such as decision trees, neural networks, and random forests on the fly. RapidMiner Studio enables the graphical user interface of the RapidMiner platform.
- Integration with popular machine learning libraries, such as TensorFlow, to build more complex models.
- Integrated evaluation metrics, such as accuracy, precision, recall, and F1 score, to evaluate the performance of a predictive model.
- Option for users to create customised evaluation metrics based on specific requirements.
- Multiple visualisation options, such as bar charts, decision trees, and scatter plots, to understand the characteristics of the data and the predictions. Users can also create custom visualisations.
2. KNIME
KNIME is a flexible and powerful open-source data analytics platform for creating predictive analytics workflows.
The key USPs of KNIME, which accounts for its soaring popularity, include:
- Tools for preprocessing, cleaning, transforming, and visualising data. The deep insights on offer make it easy to understand the structure and characteristics of the data before and after processing.
- Several nodes for building and evaluating predictive models, such as decision trees, random forests, and support vector machines. The tool also integrates with TensorFlow, scikit-learn, and other machine learning libraries to build complex predictive analytics models.
- Ready-made metrics such as accuracy, precision, and recall to evaluate the performance of built models. Users can also use KNIME’s visualisation nodes to illustrate the model performance.
3. H2O Driverless AI
H2O Driverless AI, the open-source platform, has soared in popularity recently owing to its simplicity. Even lay users can use the platform to build advanced models. This auto-ML suite automates the end-to-end process of building and deploying machine-learning models. Driverless AI, built on H2O, the open-source in-memory platform, handles large datasets.
Custom recipes enable experts and citizen data scientists to develop AI models effortlessly.
The key USPs of H2O Driverless AI include
- End-to-end automation of building, tuning and deploying machine learning models. The suite offers automated capabilities for feature engineering, model selection, natural language processing, semantic analysis, and parameter tuning.
- Simplified models using causal graphs, LIME, Shapley and decision tree surrogate methods.
- Tools for understanding machine learning models’ behaviour and insights into algorithms decisions.
- Ability to export models in several formats, including API, MOJO, Python, R, and REST). Deploying the model in any environment or format becomes easy.
- Driverless AI finds widespread application across banking, healthcare, retail, transportation, and other sectors.
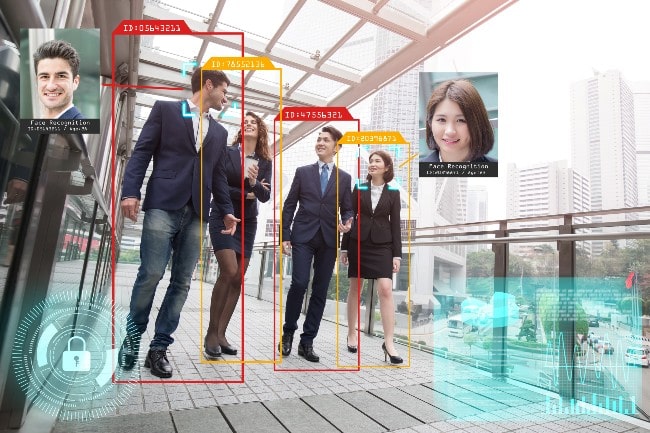
4. Alteryx
Alteryx provides an integrated, user-friendly platform for predictive analytics.
The platform converges analytics, data science and process automation into one platform. The platform offers
- Easy drag-and-drop interface
- A wide variety of pre-built data transformation and cleaning tools.
- Pre-built modelling tools, including linear and logistic regression, decision trees, and k-means clustering. Business users may use these tools to build predictive models with minimal coding.
- Ability to deploy predictive models as web services for easy consumption by other applications.
- Wide range of data visualisation tools to gain insights from data and communicate findings.
- A shared and governed environment for data analysts, business users, and data scientists to collaborate.
- Ability to deploy workflows on-the-fly using Alteryx Server or Alteryx Analytics Hub.
5. DataRobot
DataRobot, with its machine learning capabilities, is another popular predictive analytic modelling platform. The platform is scalable and can process large datasets in parallel quickly.
The key USPs include
- Automation of core steps in preparing predictive models. Users can automate model and feature selection and hyperparameter tuning to speed up the process.
- Model interpretability, or explanations on how the models work to help users understand the results better
- Pre-built models. Like most other tools, DataRobot also offers several pre-built models.
- Multiple deployment options. Users may run models as a web service, batch job, or real-time API, making it easy to integrate predictive models into business applications.
- A collaborative environment for data scientists, analysts, and business users
6. IBM Watson Studio
IBM is one of the leading names in predictive analytics after its 2009 acquisition of SPSS. The platform has retained its popularity despite challenges from newer open-source upstarts.
IBM Watson Studio offers a cloud-based integrated development environment. IBM SPSS Modeler, the data mining and text analytics software, provides a graphical user interface. Business managers may use these resources to quickly prepare data and build-deploy models.
The platform’s key USPs include:
- Integrated tools for cleansing and preparing, developing and training machine learning models, and deploying tools in a real environment. The platform co-opts several tools and services, including Apache Spark, Jupyter notebooks, and RStudio, for data preprocessing, visualisation, and modelling.
- A wide range of pre-built data mining algorithms, including decision trees, neural networks, and ensemble methods, allow developers to hit the ground running.
- Access to machine learning and deep learning libraries, such as TensorFlow and PyTorch, enables users to deploy models in various formats, such as REST APIs and Docker containers.
- Advanced text analytics capabilities ease natural language processing. Easy integrations with Watson Natural Language Understanding, Watson Assistant, and other IBM services enable adding advanced analytics and AI capabilities to projects on-the-fly.
- A ready-made deployment wizard allows users to deploy models as web services, Predictive Model Markup Language files, or R or Python scripts.
7. Microsoft Azure Machine Learning
The Azure machine learning platform sustains popularity as part of the Microsoft stable. Many business users opt for Microsoft’s Power BI analytics platform and Excel as their analytics front-end. The cloud-based Microsoft Azure Machine Learning platform adds machine learning models. The front-end tools get predictive analytics capabilities.
The Microsoft Azure Machine Learning platform offers
- Integration with Azure Data Catalog, Data Factory and HDInsight for predictive analytics capabilities. Ready-made tools take care of data cleansing, transformation, and feature engineering.
- Strong integration with application development and RPA tooling. Deploying predictive analytics capabilities directly into applications and business workflows becomes easy. The platform offers seamless integration with Azure SQL Database, Azure Blob Storage, and Azure Data Lake.
- A range of model evaluation and selection tools, including cross-validation and hyperparameter tuning.
- The Ability to deploy trained models as web services for real-time inferencing.
- Tools for tracking and managing projects, including experiment tracking and model management.
2023 will be a watershed year for data analytics and especially predictive analytics. As competition and uncertainties intensify, more businesses will adopt these tools to make better-informed decisions. The rising popularity of AutoML tools and the increased use of low-code/no-code automation will deliver prescriptive analytics directly into workflows, further expanding its popularity. But only having accessible technology does not make a difference. Competitive advantage stems from knowing how to apply technology to solve business problems.